Behavioral Analytics a technique that uses machine learning, big data, artificial intelligence, and analytics to identify patterns and anomalies in human behavior.
Instances of fraud are on the rise across all industries, putting merchants, governments, and individuals on alert to protect their sensitive data and assets. More specifically, fraud is impacting many online merchants as e-commerce shopping has grown rapidly since the onset of the pandemic in 2020.
As fraud detection efforts increase as a way to mitigate these risks, organizations are discovering new and advanced techniques to become quicker and more accurate with their fraud detection abilities.
One method these organizations are employing is through Behavioral Analytics. In doing so, leaders can familiarize themselves with the typical behaviors of fraudsters so it becomes easier to detect when malicious activities are underway.
What Is Behavioral Analytics for Fraud Detection?
Behavioral Analytics is the act of analyzing someone’s online behaviors to help detect when the activities are done by the rightful account holder, or someone posing as the individual. This method of fraud detection is based on machine learning algorithms, making it effective at detecting fraud since it continually learns from new data points rather than being built around static rules. As a result, these programs can learn the evolving methods of fraudsters that a rule-based system might not detect.
Here are some examples of such behavioral data that might be tracked for better fraud detection:
– Time of log-in
– Hesitation
– Time to enter responses
– Automated entry
The goal of Behavioral Analysis is to monitor this data to help detect when nefarious activity is underway so they can prevent instances of loss for the individual and the company itself.
When done in real-time, Behavioral Analytics is a critical tool for online organizations today when it comes to bolstering their fraud detection efforts. When fine-tuned with advanced capabilities, Behavioral Analytics adds minimal to no friction to legitimate user experience.
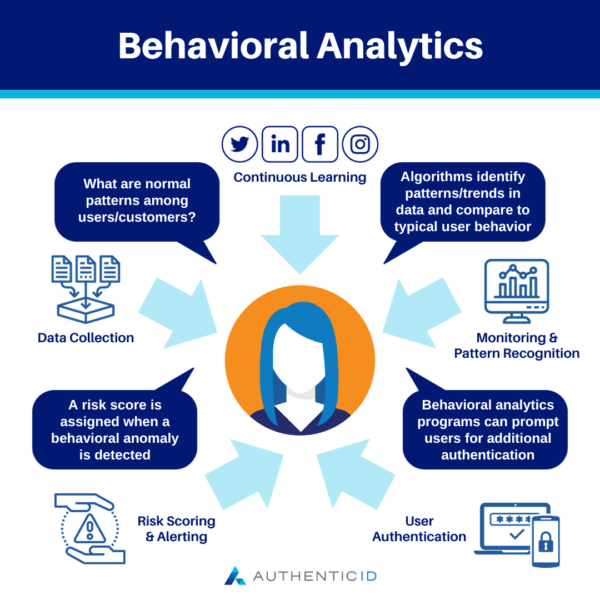
How Does Behavioral Analytics Work to Detect Fraud?
Behavioral Analytics adds an additional layer of protection that organizations can use on top of standard authentication methods like Two-Factor Authentication (2FA) or password-username log-in portals.
But, Behavioral Analytics relies on large amounts of data so companies can detect who their legitimate customers are, and who is at risk of making a fraudulent transaction. In other words, in order to detect bad actors, organizations need to analyze the behavior of actual customers to see what normal behavior patterns look like. They can use these “regular” and consistent patterns of behavior to identify deviations that may indicate fraudulent activity. In practice, here is how it might look to use Behavioral Analytics for fraud detection.
1. Data Collection:
Organizations will need to collect a wide range of data points on user behavior to start understanding what normal behavior patterns look like among their users. Some of the data that will be collected include log-in times, locations, devices used, transaction history, interactions on the website, and others.
2. Profiling Users:
After collecting data, organizations will need to create profiles for each of their customers or users. Using these profiles, they’ll be able to track the user’s historical behavior and characteristics to form a baseline of normalized behavior.
3. Monitoring & Pattern Recognition:
As we mentioned earlier, Behavioral Analytics is based on advanced machine learning algorithms to analyze the collected data. The algorithms will identify patterns, trends, and correlations in the data, and then compare them to current user behavior patterns to identify any deviations or anomalies from typical behavior.
4. Risk Scoring & Alerting:
When a behavioral anomaly is detected, Behavioral Analytics programs will assign a risk score to the activity or transaction. In general, a higher risk score will indicate that there’s a great likelihood that the behavior is fraudulent. So, if the risk score passes a certain threshold, the system will generate an alert to the security team for further review of the detected risk factors.
5. User Authentication:
In some cases, a Behavioral Analytics program can be set up to prompt users with additional authentication or verification steps when high-risk behavior is detected. This might include answering security questions or entering the PIN sent to a verification app.
6. Continuous Learning:
Behavioral Analytics programs are dynamic and adaptive, using new data collection to update their algorithms based on new inputs. So, the system can adapt and evolve to new tactics and behaviors of fraudsters. This compares to other methods of fraud detection which are rule-based and static, with any updates to the system occurring manually through administrators.
Common Use Cases of Behavioral Analytics in Fraud Detection
Behavioral Analytics can be put to use for a variety of use cases across several industries. Here are some of the common ways that Behavioral Analytics can be applied in fraud detection:
- Payment Fraud Detection: The system analyzes the behavior of users when making online transactions/payments; it will look for unusual patterns or deviations from normal patterns like unusual purchase amounts, locations, or frequency of transactions
- Account Takeover Prevention: To prevent fraudsters from gaining unauthorized access to a user’s account, Behavioral Analytics can help identify suspicious login patterns like multiple failed attempts, changes in location, or unidentified devices to prevent such attacks
- Network Security: Behavioral Analytics can be used to help support network security by detecting cyber threats or intrusions
- Insider Threat Detection: Organizations can more accurately detect insider threats by monitoring employees’ digital activities and looking for suspicious activity like data exfiltration, or unusual file access patterns that could indicate malicious intent
- Identity Theft Detection: Behavioral Analytics can be used to identify identity theft by monitoring user behavior and identifying discrepancies in their profiles or activities, like when multiple people are trying to access the same account from different locations
- E-commerce Fraud Detection: E-commerce sellers use Behavioral Analytics to prevent fraudulent chargebacks, account takeovers, and fake reviews; it can identify unusual shopping patterns, high-risk transactions, and changes in shipping or billing information
- ATM/Card Fraud Prevention: Banks and financial institutions can use Behavioral Analytics to detect when ATM or card usage is fraudulent; this might include suspicious ATM withdrawal patterns or if card usage deviates from typical behavior