Friendly fraud, also known as first-party fraud, can take many different forms, but it generally entails an actual consumer purchasing goods or services from a business and then making false claims. These claims can include the purchase or the delivery of the goods, the need for a refund as a result of the false claim, or the fact that they only received a portion of the ordered goods to keep said goods without being charged for them. Friendly Fraud was given its name because retailers believed the customer was making an honest claim. Friend Fraudsters can take many shapes, from unintentional mishaps to skilled criminals, this makes it difficult for customer service agents to identify if the claim is fraudulent or an honest mistake.
How Does Friendly Fraud Occur?
Customers who commit friendly fraud make a credit card purchase, receive the goods or service, and then request a refund for a misplaced or inaccurate order. Or they might file a chargeback with the credit card company issuing bank with the hope of getting a complete refund of the purchase price.
Friendly Fraud, also known as chargeback fraud, is estimated to have cost businesses in the United States more than $4.8 billion in 2021. Furthermore, it is estimated that up to 80% of all chargebacks could be considered fraudulent.
When a chargeback is filed fraudulently, it then falls on the merchant to pay for the “lost” merchandise, lost labor, and extra chargeback fees, and shipping fees, unless they can verify the charge was actually valid. The merchant may end up paying twice as much as the original transaction. If a merchant receives too many chargebacks, they may face more than just fees: some merchants can face a loss of their merchant accounts if too many chargebacks are filed under their business. This can be detrimental to business operations and even the livelihood of a retailer.
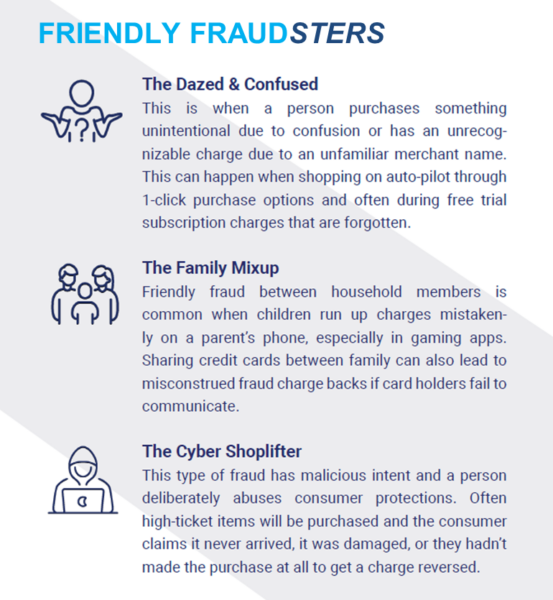
How to Identify Friendly Fraud
Identifying friendly fraud is difficult due to customer claims that appear credible. Businesses can reduce the amount of friendly fraud that occurs by implementing their tracking and shipping process. This means that the customer must sign for the product on delivery, or that the company can implement paper trails to prove product orders and delivery. Clear and precise refund policies can also help to reduce customer fraud claims based on false product descriptions. Finally, it is critical to provide a refund policy that clearly states specific guidelines for the time frame the customer has to return a product.
Friendly fraud is also extremely difficult to predict because it is usually committed by what would seem to be genuine customers. Occasionally the fraudster will only request a partial refund for a fraction of their order, or they could be a regular customer who never disputes anything but will dispute a peculiar order. It may not have been something they intended to do at all, but fraud occurs when they willfully accept a product or service they know they should be honestly paying for.
How to Prevent Friendly Fraud
As a merchant, the best way to combat friendly fraud is to prevent it from occurring in the first place. You must clarify customer satisfaction and uncertainty, which leads to friendly fraud, in order to reduce chargebacks. It is critical to track offenders and blacklist cards in order to prevent intentional fraud.
There are other preventative measures one can take to detect friendly fraud. Paying close attention to unusual or rather larger orders than your average order, seeing if a customer’s order frequency has increased unexpectedly, and you can also use customer deny lists to identify customers who are more likely to commit friendly fraud. Providing excellent customer service and simple communication tools on your e-commerce website are two ways to delight your customers and can also help decrease friendly fraud incidents.
Ultimately, the most effective way in reducing chargeback abuse is to use fraud detection technology that uses advanced machine learning algorithms as well as manual reviews by in-house risk analysts.